-
The grand challenge is focused on creating a SocialBot, an Alexa skill that converses coherently and engagingly with humans on popular topics and news.
-
The challenge is focused on helping advance development of next-gen virtual assistants that will assist humans in completing real-world tasks by continuously learning, and gaining the ability to perform commonsense reasoning.
-
The challenge is focused on developing agents that assist customers in completing complex tasks that require multiple steps and decisions. It's the first conversational AI challenge to incorporate multimodal (voice and vision) customer experiences.
Resources
-
View the competing Alexa Prize teams from universities around the world, and learn more about the students, team leaders, and faculty advisors.
-
See the research in conversational AI resulting from the pursuit of the Alexa Prize competition goals.
-
Before getting in touch, check and see if we've covered your query in our frequently asked questions.
-
Teams build their bots using the Alexa Skills Kit (ASK), which enables them to receive continuous feedback on their inventions in real-world settings.
Up next
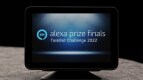
Alexa Prize TaskBot Challenge Finals
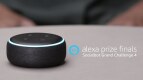
Alexa Prize SocialBot Grand Challenge 4
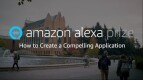
How to create a compelling Alexa Prize application
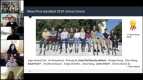
Panelists discuss the Alexa Prize during WSDM 2021
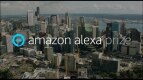
Welcome to the Alexa Prize
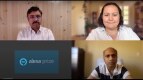
Winners of the Alexa Prize SocialBot Grand Challenge 3 discuss their research
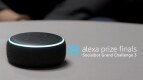
Alexa Prize SocialBot Grand Challenge 3
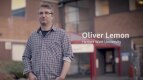
Understanding conversational AI with Professor Oliver Lemon
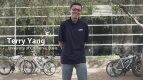
Team Gunrock, UC Davis, discuss the Alexa Prize SocialBot Grand Challenge 3
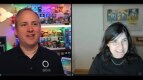
Yoelle Maarek, Alexa Shopping vice president of research and science
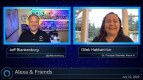
Dilek Hakkani-Tür, Alexa AI senior principal scientist
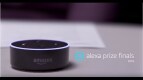
Alexa Prize SocialBot Grand Challenge 2
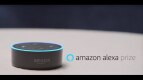
Alexa Prize SocialBot Grand Challenge 1
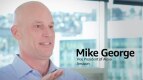
Introducing the Alexa Prize
Alexa Prize TaskBot Challenge Finals
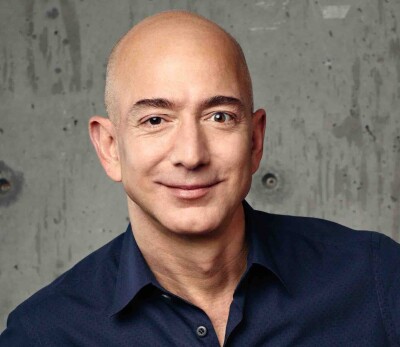
I believe the dreamers come first, and the builders come second. A lot of the dreamers are science fiction authors, they’re artists...They invent these ideas, and they get catalogued as impossible. And we find out later, well, maybe it’s not impossible. Things that seem impossible if we work them the right way for long enough, sometimes for multiple generations, they become possible.
Jeff Bezos, founder and executive chairman of Amazon